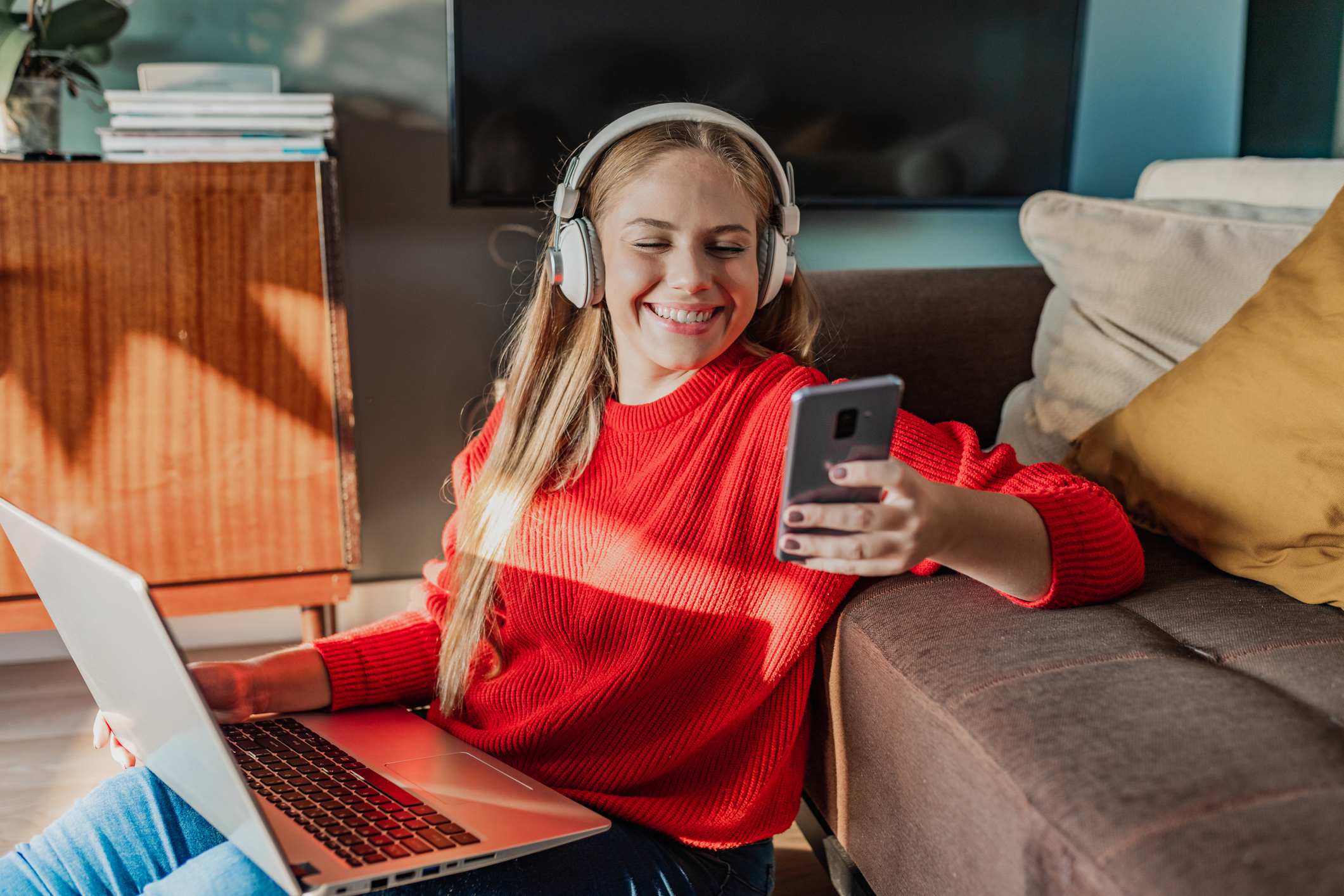
Insights
Analysis, advice and inspiration. Sharing what we’ve learned with each other, our clients and our coding community expands our collective capabilities. Knowledge empowers.
Analysis, advice and inspiration. Sharing what we’ve learned with each other, our clients and our coding community expands our collective capabilities. Knowledge empowers.
Digital community
Insight, imagination and expertly engineered solutions to accelerate and sustain progress.
Contact